I’m Yuko Suzuki, a PhD student at the Institute of Educational Technology at the Open University in the UK.
My research entitled ‘Making Science More comprehensible with the Use of Extra Dimensions’ is something to explore how mixed reality technologies, including 3D CAD models, 3D animations, AR and VR, can make the scientific knowledge, the concepts of modern physics in particular, more comprehensible and efficiently deliverable at cognitive levels. On this page is my initial research proposal written in 2020.
For the latest of the research project, please click the image below. You can see the progress overview.
If you are interested in collaborating with me, please feel free to get in touch!
Introduction
Technology can help make the learning process easier and therefore science more comprehensible. According to Fullan, the integration of technology, pedagogy, and change knowledge could be designed to create learning experiences that increase engagement and efficiency in what is learned (2013).
In this research, I would like to explore how content creators, including broadcasters, digital producers and educational bodies, can develop more effective science learning content and visual tools to increase the public engagement with science, physics in particular, and to extend their knowledge more efficiently, using extra dimensions i.e., 3D modelling and animation techniques, based on cognitive theories, theories of visualisation, and social constructivism.
My hope is that this research could help a wider audience from different backgrounds engage in science learning in diverse educational environments and keep pace with the accelerated scientific and technological developments of today.
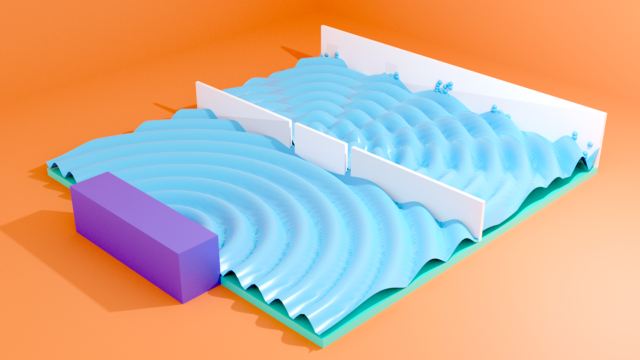
Background/Literature Review
Science, particularly physics and chemistry, has been seen as among the most difficult subjects by students for many decades (Duckworth & Entwistle, 1974; Tripney et al., 2010). To overcome this stigma, professional content producers have been putting much effort in the realm of enjoyment of science. Television science programmes often represent science as spectacular and awe-inspiring, and its landscape is changing from the traditional science documentary to contemporary factual entertainment (Campbell, 2016).
On the other hand, on the digital platforms such as YouTube, where people consume a significant number of science videos produced by a broad range of creators, the motivation of viewing activities is characterised by both positive affective states and goal-related cognitions (Krapp & Prenzel, 2011; Lin et al., 2013). Recent studies show that individuals engage with online science videos in order to satisfy their learning goals (Moll & Nielsen, 2017; Allgaier, 2019). Watching science videos is now lifelong, free-choice, self-directed science learning (Rosenthal, 2018; Falk et al., 2016).
The framework to investigate effectiveness of science content has been developed mostly in the field of multimedia learning in the effort to transform traditional methods to more efficient ones (Mayer, 2005; Clark, 2007). Cognitive Load Theory (CLT) is a commonly used established study reference and has made influential suggestions (Martin, 2015; Anmarkrud et al., 2019; Mutlu-Bayraktar et al., 2019).
According to CLT, information is held in working memory during learning, while being processed sufficiently, before it’s passed into long-term memory. Due to the limited capacity of working memory, when it is overloaded, learners’ information processing slows down (Paas & Sweller, 2012). Therefore, the cognitive load needs to be managed in order to make the learning process more efficient (van Merriënboer & Sweller, 2005; Clark & Mayer, 2016).
CLT identifies three types of cognitive load: 1) intrinsic cognitive load, that is indigenous to the material dealt with, 2) extraneous cognitive load, that is unnecessary for learning, and 3) germane cognitive load that contributes to organisation and integration of information (Paas et al., 2003). Extraneous load and germane load can be intervened by instructional design (Sweller et al., 1998).
Based on CLT, recent studies developed augmented reality (AR) learning tools and researched their effectiveness (Wu et al., 2018; Lai et al., 2018; Thees et al., 2020). Assuming that the challenge for learners is to capture the link between experimentation and the corresponding theoretical background, researchers conducted the experiment to support learners to visualise such links using AR technology. They reported a significant reduction of extraneous load for AR-based workflow regardless of the increase of sensory information (Thees et al., 2020).
The effectiveness of 3D representations has been also investigated in respect to various learning tools (Vivou, 2002; Gosalia et al.,2015; Wu & Shah, 2004). Research concluded that 3D representations help students overcome conceptual errors related to visuospatial operations (Wu & Shah, 2004).
Another study reported that 3D illustration has an advantage over 3D animation in reducing cognitive load while 3D animations do increase the interest of students and make the material more appealing to them (Korakakis et al., 2008). On the other hand, a more recent study confirmed the effectiveness of 3D animation created with a newer technology through the subjective evaluation method (Nasir et al., 2018), but they did not use the framework of CLT.
With regard to learners’ interest, many studies have investigated the seductive details effect and remarked that they can arouse motivation and attraction of students (Wang & Adesope, 2016). However, other research reported that seductive details could be detrimental in learning outcomes (Mayer, 1993). It seems that the balance between the effect of motivation and cognitive loads needs to be met.
Gilbert differentiates visualisation into two: internal visual representations referred as mental images and external representations open to the public (Gilbert, 2008). While internal representations are converted into external ones, and vice versa, particularly in communication, they don’t always coincide with each other (Rapp & Kurby, 2008).
Further, internal representations are divided into three types of form: the macro type, that can be investigated with instruments, the sub-macro type, that is too small to be seen with an optical microscope, and the symbolic type, that is represented with signs and letters (Gilbert 2008). The understanding of scientific phenomena involves the ability to construct and navigate between these different forms, and it requires three-dimensional operations. This is identified as a major challenge for students (Wu & Shah, 2004; Gilbert, 2010).
In fact, digital content increasingly uses 3D animation to visualise the abstract concepts of physics (3Blue1Brown; Khutoryansky), presumably trying to bridge the gap between these different forms and making a closer connection between the external representations and the perception of real world. However, simpler 2D explanations still remain equally popular (Minute Physics; Looking Glass Universe).
Visualisation is not limited as a tool to support conceptual understanding. The recent approach is to position visual representations as epistemic objects to make science learning closer to the reality of scientific practice (Evagorou et al., 2015). This is similar to the science documentary approach, and 3D and animation techniques allow more possibilities of incorporating the practice of science in learning materials.
From the perspective of social constructivism, which suggest that knowledge is formed in the interaction with others (Vygotsky et al., 1978; Bada, 2015), these visual representations on digital platforms can be seen as scaffolding to aid learning (Roehler & Cantlon, 1997), produced and developed by more experienced peers in dialogue with learners.
While scaffolding should work in the zone of proximal development (ZPD), where learners advance to a higher level beyond their current ability (Jie et al., 2020), the cognitive constructivism suggests that each learner’s cognitive process of knowledge acquisition is different (Powell & Kalina, 2009). Although a pool of varied content could offer learning tools corresponding to a wide range of learners’ ZPD, selecting an adequate one could be quite cumbersome. Thus, more personalised AI-based approach, such as intelligent tutoring systems, is being explored to model the ZPD and personalise the experience (Chounta et al., 2017; Witschel et al., 2020). But the recent research focuses on natural language rather than visualisation.
Now social constructivist view that scientific knowledge originates in the social world rather than the natural world is seen as key for purposeful science learning (Park & Song, 2019), and more meaningful usage of digital technologies based on this view is called for in science teaching (Barak, 2016; Jie et al., 2020).
Few studies made an extensive analysis of dimensionally different visual representations and their roles and designs in regard to relatively complex and abstract concept in physics. To maximise the effectiveness of content created with the latest technologies, rigorous research that takes cognitive loads, visuospatial capability and social constructivism into consideration is needed.
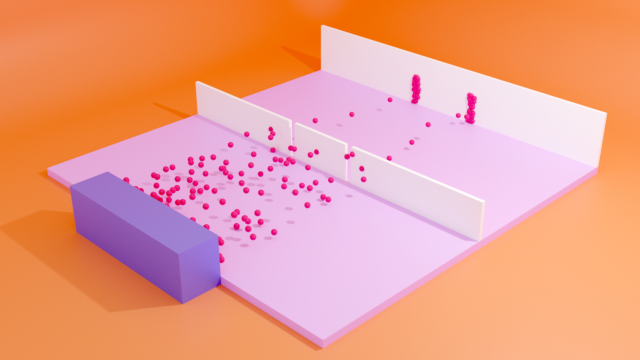
Objectives
The objective of this research is to identify how the efficiency and effectiveness of science content can be improved using the latest digital technologies that allow us to produce various visual representations. Clark et al. defined efficiency as greater learning outcome and less mental effort (2011). As many of media content are produced without a standard of viewers’ prior knowledge (Clark & Mayer, 2016), content that can establish relevant background information quickly would also be ideal.
A study reported that the numbers of physics materials on digital platforms are much smaller compared to biology counterparts (Nizaar et al., 2020). Thus, the current research will examine physics content for the future development. In addition, because some of the concepts of modern physics are often abstract and more difficult to perceive in the everyday sense, it is beneficial to come up with an easier way to understand this subject.
Previous studies assessed and made suggestions on the use of picture, diagrams, graphics, text, narration and animation, and more recently Virtual Reality (VR) and AR (Mayer & Moreno, 2003; Mutlu-Bayraktar et al., 2019; Tindall-Ford, 2019). In this research, the focus will be on the following types of representations as advanced 3D animation is gaining popularity on digital platforms, and production technology is getting more prevalent.
- 2D illustration
- 2D animation
- 3D illustration
- 3D animation
- self-operative 3D model
Although this research could be extended to VR, for the reason why the penetration rate of VR ready devices is much smaller than that of the devices which allow us to use other forms (Tankovska, 2020), the investigation on VR will be excluded for now.
Several hypotheses can be made about different types of representations:
- Animation can present visual representations of changing physical states, and, in theory, 24-60 times more images to viewers per second because of the standard frame rate. This could make animation more efficient than illustrations as learners can attend to more organised information in a shorter period of time.
- 3D representations could aid to enhance spatial visualisation, therefore, to help manage germane cognitive load, as they are a more natural and realistic depiction of our world and many of physical phenomena are described in the function of space coordinate x, y, z and time t.
- 3D and animation could help combine two kinds of visual representations: epistemic objects and conceptual explanations, bringing science learning closer to the real-world practice of science (Evagorou et al., 2015), and cover a wider spot of the ZPD.
- The amount of sensory information given inevitably increases as the dimension of content increases. Therefore, 3D illustration has an advantage over 3D animation in terms of cognitive load (Korakakis et al. 2008), and presumably 2D animation would have an advantage over 3D animation.
- Extraneous load and germane load can be intervened by instructional design (Sweller et al., 1998). Therefore, 3D and animation content could be designed in the way that cognitive loads are managed, just like AR helped reduce cognitive loads in the experiment despite the increase of sensory information (Thees et al., 2020).
- Self-operative 3D models could help manage the cognitive load and the ZPD by allowing individuals to learn at their own pace (Clark & Mayer, 2016).
- The norm of mental images about long-existing scientific concepts might be evolving as a result of interaction with others and the exchange of external representations on digital platforms.
I will attempt to identify to what extent learners can cope with the increased information at a cognitive level, and to see whether a particular representation is more suited than the other in learning a certain concept or knowledge. I will also research how visualisation could evolve and impact our mental images and notion of the physical world with social interactions on digital platforms.
Methodology
First of all, online science content produced by various creators on digital platforms such as YouTube, BBC iPlayer, and Instagram will be collected and analysed. They can be seen as a collective of external representations of people’s mental images. By taking samples to cover a certain theory or a phenomenon of modern physics, e.g., the equivalence of mass and energy, the wave-particle duality, and quantum entanglement etc. in different representation forms, its components will be classified. Then the elements and features in common among highly rated content will be identified.
Audience perception and level of comprehension can be evaluated from the comments and likes/dislikes posted for an individual video if it is on social media platforms. Online surveys in the form of questionnaire, with both lay audience and those who studied physics, can be conducted to find out which representations are closer to their mental images and which features makes learning of the target knowledge easier.
Considering these features, sample content will be prepared for an experiment with subjects to make a comparison among various representations. Different versions of content will be created for each to-be-learned theory or knowledge of physics using Adobe Creative Cloud and/or open-source application blender.
Preliminary tests will be run with the subject method. The subjective method includes pre-test and post-test questionnaires, personal interviews, and quizzes related to the theory covered in the content samples. If cognitive overload and/or visuospatial issues are detected in any version of sample content, its components will be analysed and tweaked to prepare refined versions.
The final test will be implemented with both subjective and objective methods. As for the objective method, the eye-tracking method will be used as this is an established objective method adapted in the field (Mayer, 2005; Pouw et al., 2016; Korbach et al., 2017).
Studies show that blink activity, pupil size, and fixation duration and rate all correlate significantly with different levels of demand on working memory. Blink latency, pupil size, and fixation duration progressively increases with task difficulty while blink rate, fixation rate, and saccade speed and size decreases. The advantage of this method is that we can collect real time data (Martin, 2015), which could be reflected along the timeline of the sample content.
Research participants will be recruited through personal contacts, social media and relevant websites with full compliance to research ethics, including informed consent, privacy protection, limited data usage, anonymity, and confidentiality. Only characteristics of participants and the data relevant to the research purposes will be recorded and used for analysis.
Timeline
In the first year, online science content suitable for research purposes will be gathered, and the components and features behind their effectiveness will be analysed. Based on the features identified from the analysis of existing content and the applicable theories from literature review, sample content for the experiment will be prepared.
In the second year, the preliminary experiments with prepared content will be conducted, and the components that cause cognitive overload and visuospatial issues will be analysed. Then, refined versions of sample content will be prepared, and its effectiveness will be tested.
In the final year, the analysis of existing content and the experimental results will be written up. I would like to come up with the ideal version of content with the latest technologies and make suggestions on how we could improve the efficiency and effectiveness of science learning using extra dimensions for future development in diverse learning environments.
(2480 words)
Bibliography
Anmarkrud, Ø., Andresen. A., & Bråten. I. (2019). Cognitive Load and Working Memory in Multimedia Learning: Conceptual and Measurement Issues. Educational Psychologist 54(2). https://doi.org/10.1080/00461520.2018.1554484
Allgaier, J (2019). Science and Medicine on YouTube, Second International Handbook of Internet Research 7-27
Bada, S. O. (2015). Constructivism Learning Theory: A Paradigm for Teaching and Learning. IOSR Journal of Research & Method in Education, Vol. 5, 6(1), 66-70.
Barak, M. (2016). Science Teacher Education in the Twenty-First Century: A Pedagogical Framework for Technology-Integrated Social Constructivism. Research In Science Education, 47(2), 283-303. https://doi:10.1007/s11165-015-9501-y
Campbell, V. (2016). Science, Entertainment and Television Documentary. Palgrave Macmillan.
Chounta, I., McLaren, B., Albacete, P., Jordan, P. & Katz, S. (2017). Modeling the Zone of Proximal Development with a Computational Approach. Proceedings of the 10th International Conference on Educational Data Mining (EDM 2017), 56-57.
Clark, R. C. (2007). Developing technical training: A Structured Approach for Developing Classroom and Computer-based Instructional Materials, 3rd Edition. Pfeiffer
Clark, R. C., & Mayer, R. E. (2016). E-learning and the science of instruction: Proven guidelines for consumers and designers of multimedia learning. Wiley.
Clark, R. C., Nguyen, F., & Sweller, J. (2011) Efficiency in learning: Evidence-based guidelines to manage cognitive load. Wiley.
Duckworth, D., & Entwistle, N. J. (1974). Attitudes to school subjects. British Journal of Educational Psychology, 44, 76–83. https://doi.org/10.1111/j.2044-8279.1974.tb00769.x
Evagorou, M., Erduran, S., & Mäntylä, T. (2015). The role of visual representations in scientific practices: from conceptual understanding and knowledge generation to ‘seeing ’how science works. International Journal of STEM Education, 2(1). https://doi.org/10.1186/s40594-015-0024-x
Falk, J. H., Dierking, L. D., Swanger, L. P., Staus, N., Back, M., Barriault, C., and Verheyden, P. (2016). Correlating science center use with adult science literacy: An international, crossinstitutional study. Science Education, 100(5), 849-876.
Fullan, M. (2013). Pedagogy and change: Essence as easy. Stratosphere, 17-32. Pearson.
Gilbert, J. K. (2008). Visualization: An Emergent Field of Practice and Enquiry in Science Education. Visualization: Theory and Practice in Science Education. Springer, Dordrecht. https://doi.org/10.1007/978-1-4020-5267-5
Gilbert, J. K. (2010). The role of visual representations in the learning and teaching of science. Asia-Pacific Forum on Science Learning and Teaching, 11(1).
Gosalia, N. (2015). 3D Gesture-Recognition Based Animation Game. Procedia Computer Science 45, 712 – 717. https://doi.org/10.1016/j.procs.2015.03.138
Jie, Z., Sunze, Y., & Puteh, M. (2020). Research on Teacher’s Role of Mobile Pedagogy Guided by the Zone of Proximal Development. Proceedings of the 2020 9th International Conference on Educational and Information Technology (ICEIT 2020). Association for Computing Machinery, 219–222. https://doi.org/10.1145/3383923.3383965
Korakakis, G., Pavlatou, E. A., Palyvos, J. A., N, Spyrellis. (2008). 3D visualization types in multimedia applications for science learning: A case study for 8th grade students in Greece. Computers & Education, 52(2), 390-401. https://doi.org/10.1016/j.compedu.2008.09.011
Korbach, A., Brünken, R. & Park, B. (2017). Measurement of cognitive load in multimedia learning: a comparison of different objective measures. Instructional Science, 45, 515–536. https://doi.org/10.1007/s11251-017-9413-5
Krapp, A., & Prenzel, M. (2011). Research on interest in science: Theories, methods, and findings. International Journal of Science Education, 33(1), 27-50. https://doi.org/10.1080/09500693.2010.518645
Lai, A., C Chen., & Lee, G. (2018). An augmented reality‐based learning approach to enhancing students’ science reading performances from the perspective of the cognitive load theory. British Journal of Education Technology, 50(1). https://doi.org/10.1111/bjet.12716
Lin, H. S., Lawrenz, F., Lin, S. F., & Hong, Z. R. (2013). Relationships among affective factors and preferred engagement in science-related activities. Public Understanding of Science, 22(8), 941-954. https://doi.org/10.1177/0963662511429412
Martin, S. (2015). Measuring cognitive load and cognition: metrics for technology-enhanced learning. Educational Research and Evaluation, 20(7-8), 592-621. https://doi.org/10.1080/13803611.2014.997140
Mayer, R. E. (1993). Illustrations that instruct. In R. Glaser (Ed.), Advances in instructional psychology, 5, 253–284. https://doi.org/10.4324/9781315864341-4
Mayer, R. E. (2005). The Cambridge Handbook of Multimedia Learning. Cambridge University Press
Mayer, R. E. & Moreno, R. (2003). Nine Ways to Reduce Cognitive Load in Multimedia Learning. Educational Psychologist, 38(1), 43–52. https://doi.org/10.1207/S15326985EP3801_6
Moll, R, & Nielsen, W. (2017). Development and validation of a social media and science learning survey, International Journal of Science Education, Part B, 7:1, 14-30. https://doi.org/10.1080/21548455.2016.1161255
Mutlu-Bayraktar, D., Cosgunb, V., & Altan, T. (2019). Cognitive load in multimedia learning environments: A systematic review. Computer and Education, 141. https://doi.org/10.1016/j.compedu.2019.103618
Nasir, M., Prastowo R. B. & Riwayani. (2018). An analysis of Instructional Design and Evaluation of Physics Learning Media of Three Dimensional Animation Using Blender Application. 2nd International Conference on Electrical Engineering and Informatics, 36-41. https://doi.org/10.1109/ICon-EEI.2018.8784309.
Nizaar, M., Haifaturrahmah, H. & Maryati, Y. (2020). Science Learning through YouTube Channell. Proceding International Webinar on Education.
Paas, F., Renkl, A., & Sweller, J. (2003). Cognitive load theory and instructional design: Recent developments. Educational Psychology, 38(1), 1-4. https://doi.org/10.1207/S15326985EP3801_1
Paas, F., & Sweller, J. (2012). An Evolutionary Upgrade of Cognitive Load Theory: Using the Human Motor System and Collaboration to Support the Learning of Complex Cognitive Tasks. Educational Psychology Review 24, 27–45. https://doi.org/10.1007/s10648-011-9179-2
Park, W., & Song, J. (2019). Between realism and constructivism: A sketch of pluralism for science education, In: Herring, E., Jones, K. M., Kiprijanov, K. S., & Sellers, L. M. (Eds), The Past, Present, and Future of Integrated History and Philosophy of Science. Routledge
Pouw, W. T., Mavilidi, M. F., van Gog, T., & Paas, F. (2016). Gesturing during mental problem solving reduces eye movements, especially for individuals with lower visual working memory capacity. Cognitive Processing 17, 269–277. https://doi.org/10.1007/s10339-016-0757-6
Powell, K., & Kalina, C. J. (2009). Cognitive and Social Constructivism: Developing Tools for an Effective Classroom. Education 3-13(130), 241-250.
Rapp, D. N., & Kurby, C. A. (2008). The ‘Ins’ and ‘Outs’ of Learning: Internal Representations and External Visualizations. In: Gilbert J.K., Reiner M., Nakhleh M. (eds) Visualization: Theory and Practice in Science Education. Models and Modeling in Science Education. Springer, Dordrecht. https://doi.org/10.1007/978-1-4020-5267-5_2
Roehler, L. R., & Cantlon, D. J. (1997). Scaffolding: A powerful tool in social constructivist classrooms. In: K. Hogan & M. Pressley (Eds.), Advances in learning & teaching. Scaffolding student learning: Instructional approaches and issues, 6–42. Brookline Books.
Rosenthal, S. (2018). Motivations to seek science videos on YouTube: free-choice learning in a connected society. International Journal of Science Education, Part B: Communication and Public Engagement, 8(1), 22-39. https://doi.org/10.1080/21548455.2017.1371357
Sweller, J., van Merriënboer, J., & Paas., F. (1998). Cognitive Architecture and Instructional Design. Educational Psychology Review, 10(3), 251–296. https://doi.org/10.1023/A:1022193728205
Tankovska, H. (2020). Access to a VR device in households worldwide 2020. Statista.
Thees, M., Kappa, S., Strzys, M. P., Beil, F., Lukowicz, P., & Kuhn, J. (2020). Effects of augmented reality on learning and cognitive load in university physics laboratory courses. Computers in Human Behavior, 108. https://doi.org/10.1016/j.chb.2020.106316
Tindall-Ford, S., Agostinho, S & Sweller, J. (2019). Advances in Cognitive Load Theory: Rethinking Teaching. Routledge.
Tripney, J., Newman, M., Bangpan, M., Niza, C., Mackintosh, M., & Sinclair, J. (2010). Factors influencing young people (aged 14–19) in education about STEM subject choices. A Systematic Review of the UK Literature.
Van Merriënboer, J., Sweller, J. (2005). Cognitive load theory and complex learning: Recent developments and future directions. Educational Psychology Review, 17(2), 147-177. https://doi.org/10.1007/s10648-005-3951-0
Vygotsky, L., Cole, M., John-Steiner, V., & Scribner, S. (1978). Mind in society. London: Harvard University Press.
Vivou, M. (2002). VR-ENGAGE: A Virtual Reality Educational Game That Incorporates Intelligence International Conference on Advanced Learning Technologies September 16(19), 425-430.
Wang, Z. & Adesope, O. (2016). Exploring the effects of seductive details with the 4-phase model of interest. Learning and Motivation, 55, 65-77. https://doi.org/10.1016/j.lmot.2016.06.003.
Witschel H.F., Diwanji P., Hinkelmann K. (2020). A Dialog-Based Tutoring System for Project-Based Learning in Information Systems Education. In: Dornberger, R. (eds) New Trends in Business Information Systems and Technology. Studies in Systems, Decision and Control, vol 294. Springer. https://doi.org/10.1007/978-3-030-48332-6_6
Wu, H., & Shah, P. (2004). Exploring visuospatial thinking in chemistry learning. Science Education, 88(3), 465-492. https://doi.org/10.1002/sce.10126
Wu, P., Hwang, G., Yang, M., & Chen., C. (2018). Impacts of integrating the repertory grid into an augmented reality-based learning design on students’ learning achievements, cognitive load and degree of satisfaction. Interactive Learning Environments, 26(2), 221-234. https://doi.org/10.1080/10494820.2017.1294608
3Blue1Brown YouTube channel
https://www.youtube.com/channel/UCYO_jab_esuFRV4b17AJtAw
Physics Videos by Eugene Khutoryansky YouTube channel
https://www.youtube.com/channel/UCJ0yBou72Lz9fqeMXh9mkog
Minute Physics YouTube channel
https://www.youtube.com/user/minutephysics
Looking Glass Universe YouTube channel
https://www.youtube.com/user/LookingGlassUniverse